Better data can lead to better outcomes. But what do we mean by ‘data quality’? ODI Associate Leigh Dodds, who is working with ODI Partner Experian to explore data quality in UK open datasets, explains
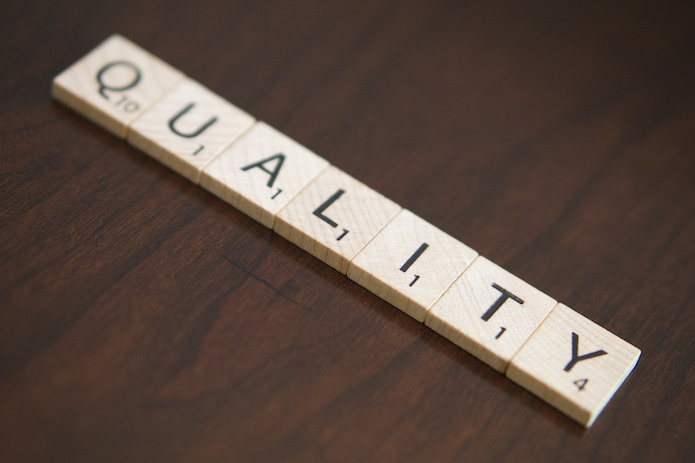
1. A quality dataset is a well-published dataset
First impressions are everything. The efforts made to publish a dataset will guide a user’s experience in finding, accessing and using it. No matter how good the contents of your dataset, if it is not clearly documented, well-structured and easily accessible, then it won’t get used.
Open data certificates are a mark of quality and trust for open data. They measure the legal, technical, practical and social aspects of publishing data. Creating and publishing a certificate will help a publisher build confidence in their data. Open data certificates complement the five star scheme, that assesses how well data is integrated with the web.
2. A dataset can contain a variety of problems
Data quality also relates to the contents of a dataset. Data errors usually occur when the data was originally collected. But the problems may only become apparent once a user begins working with the data.
There are a number of different types of data quality problem. The following list isn’t exhaustive but includes some of the most common:
- The dataset isn’t valid when compared to its schema, for example there are missing columns, or they are in the wrong order
- The dataset contains invalid or incorrect values, for example numbers that are not within their expected range, text where there should be numbers, spelling mistakes or invalid phone numbers
- The dataset has missing data from some fields or the dataset doesn’t include all of the available data – some addresses in a dataset might be missing their postcode, for example
- The data may have precision problems — these may be due to limits in accuracy of the sensors or other devices (such as GPS devices) that were used to record the data, or they many be due to simple rounding errors introduced during analysis
3. There are several ways to fix data errors
Some types of error are more easily discovered and fixed than others. Tools like CSVLint can use a schema to validate a dataset, applying rules to confirm that data values are valid. But sometimes extra steps are needed to confirm whether a value is correct.
For example, an email address (for contacting a company, for example) might be formatted correctly but it might contain a spelling mistake that means it is unusable. There are a variety of ways to improve confidence that an email address is valid, but you can only reliably confirm that an email address is both valid and actually in use by sending an email and asking a user to confirm receipt.
Another way to help identify data quality issues is to check data against a register that provides a master list of legal values. For example, country names might be validated against a standard register of countries. Open registers are an important part of the data ecosystem.
Other types of errors are much harder to fix. Company names and addresses may become invalid or incorrect over time. Publishing data openly can allow others to identify and contribute fixes. Making things open can help make them better.
4. Sometimes ‘good quality’ depends on your needs
One way to help improve data quality is to generate quality metrics for a dataset. Metrics can help summarise the kinds of issues found in a dataset. You might choose to count the numbers of valid and invalid values in specific columns. Run regularly, metrics can identify if the quality of a dataset is changing over time.
However, it’s hard to make an objective assessment about whether a dataset is of a good quality. Sometimes quality is in the eye of the beholder. For example:
- GPS accuracy in a dataset might not be important if you only want to do a simple geographic visualisation. But if you’re involved in a boundary dispute then precision may be vital.
- Inaccurate readings from a broken sensor might be an annoyance for the majority of users who might want them filtered out of a raw dataset. But if you are interested in gathering analytics on sensor failures then seeing the errors is important.
Fixing all data quality issues in a dataset can involve significant investment, sometimes with diminishing returns. Data publishers and users need to decide how good is good enough, based on their individual needs and resources.
However, by opening data and letting others contribute fixes, we can spread the cost of maintaining data. Making things open can help make them better, remember?
[This story first appeared on the Open Data Institute blog]